Home | Slides | Videos | Books/Fundamental Papers/Surveys | Workshops and Conferences | Recent Publications | Our Research Areas | Theses | Packages/Libraries
Recent publications
- Bax, K., Sahin, Ö., Czado, C., and Paterlini, S. (2023)
ESG, Risk, and (tail) dependence
International Review of Financial Analysis
[link] - Czado, C., Bax, K., Sahin, Ö., Nagler, T., Min, A., and Paterlini, S. (2023)
Vine copula based dependence modeling in sustainable finance
The Journal of Finance and Data Science
[link] - Sahin, Ö. and Czado, C. (2022)
High-dimensional sparse vine copula regression with application to genomic prediction
[preprint] - Sommer, E., Bax, K., and Czado, C. (2022)
Vine Copula based portfolio level conditional risk measure forecasting
[preprint] - Ye, F., Ding, J., Chen, K. and Xi, X. (2022)
Investigation of Corticomuscular Functional Coupling during Hand Movements Using Vine Copula
Brain Sciences
[link] - Alnasser, H. and Czado, C. (2022)
An Application of D-vine Regression for the Identification of Risky Flights in Runway Overrun
[preprint] - Tepegjozova, M. and Czado, C. (2022)
Bivariate vine copula based quantile regression
[preprint] -
Kreuzer, A., Dalla Valle, L., and Czado, C. (2022)
A Bayesian Non-linear State Space Copula Model to Predict Air Pollution in Beijing
Journal of the Royal Statistical Society: Series C (Applied Statistics)
[link] -
Sahin, Ö. and Czado, C. (2022)
Vine copula mixture models and clustering for non-Gaussian data
Econometrics and Statistics
[preprint | link ] - Tepegjozova, M., Zhou, J., Claeskens, G. and Czado, C. (2022)
Nonparametric C- and D-vine based quantile regression
Dependence Modeling
[preprint | link ] - Nagler, T., Krüger, D. and Min, A. (2022)
Stationary vine copula models for multivariate time series
Journal of Econometrics
[preprint, link] - Yang, L. and Czado, C. (2022)
Two‐Part D‐Vine Copula Models for Longitudinal Insurance Claim Data
Scandinavian Journal of Statistics
[link] - Czado, C. and Nagler, T. (2022)
Vine Copula Based Modeling
Annual Review of Statistics and Its Application, 9, 453-477
[link ] - Czado, C. and Scharl, S. (2021)
Analysis of an interventional protein experiment using a vine copula based structural equation model
[preprint] - Kielmann, J., Manner, H. and Min, A. (2021)
Stock market returns and oil price shocks: A CoVaR analysis based on dynamic vine copula models
[link] - Coblenz, M. (2021)
MATVines: A vine copula package for MATLAB
[link] - Deng, Y. and Chaganty, N.R. (2021)
Pair-copula Models for Analyzing Family Data.
J Stat Theory Pract 15, 13.
[link][access] - Chang, B. and Joe, H. (2020)
Copula diagnostics for asymmetries and conditional dependence.
Journal of Applied Statistics, 47(9), 1587-1616.
[link] - Zhu, K., Kurowicka, D. and Nane, G. F. (2020)
Common sampling orders of regular vines with application to model selection
Computational Statistics & Data Analysis
[link]
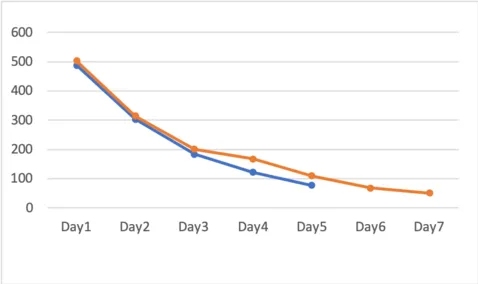
-
Alnasser, H. and Czado, C. (2022)
An Application of D-vine Regression for the Identification of Risky Flights in Runway Overrun
[preprint] -
Höhndorf, L., Czado, C., Bian, H., Kneer, J. and Holzapfel, F. (2017).
Statistical modeling of dependence structures of operational flight data measurements not fulfilling the iid condition.
In AIAA Atmospheric Flight Mechanics Conference (p. 3395).
[link] -
Hu, Z. and Mahadevan, S. (2017).
Time-dependent reliability analysis using a Vine-ARMA load model.
ASCE-ASME Journal of Risk and Uncertainty in Engineering Systems, Part B: Mechanical Engineering, 3(1), 011007.
[link] -
Schepsmeier, U. and Czado, C. (2016).
Dependence modelling with regular vine copula models: a case-study for car crash simulation data.
Journal of the Royal Statistical Society: Series C (Applied Statistics), 65(3), 415–429.
[link] -
Jiang, C., Zhang, W., Han, X., Ni, B. and Song, L. (2015).
A vine-copula-based reliability analysis method for structures with multidimensional correlation.
Journal of Mechanical Design, 137(6), 061405.
[link]

- Bax, K., Sahin, Ö., Czado, C., and Paterlini, S. (2023)
ESG, Risk, and (tail) dependence
International Review of Financial Analysis
[link]
- Czado, C., Bax, K., Sahin, Ö., Nagler, T., Min, A., and Paterlini, S. (2023)
Vine copula based dependence modeling in sustainable finance
The Journal of Finance and Data Science
[link]
-
Sommer, E., Bax, K., and Czado, C. (2022)
Vine Copula based portfolio level conditional risk measure forecasting
[preprint] -
Yang, L. and Czado, C. (2022)
Two‐Part D‐Vine Copula Models for Longitudinal Insurance Claim Data
Scandinavian Journal of Statistics
[link] -
Kielmann, J., Manner, H. and Min, A. (2021)
Stock market returns and oil price shocks: A CoVaR analysis based on dynamic vine copula models
Empir Econ (2021).
[link] -
Brechmann, E. C., Heiden, M. and Okhrin, Y. (2018).
A multivariate volatility vine copula model.
Econometric Reviews, 37(4), 281–308.
[link] -
Barthel, N., Czado, C. and Okhrin, Y. (2018).
A partial correlation vine based approach for modeling and forecasting multivariate volatility time-series.
Computational Statistics and Data Analysis, 142, 106810.
[link] -
Shi, P. and Yang, L. (2018).
Pair copula constructions for insurance experience rating.
Journal of the American Statistical Association, 113(521), 122–133.
[link] -
Aas, K. (2016).
Pair-copula constructions for financial applications: A review.
Econometrics, 4(4), 43.
[link]
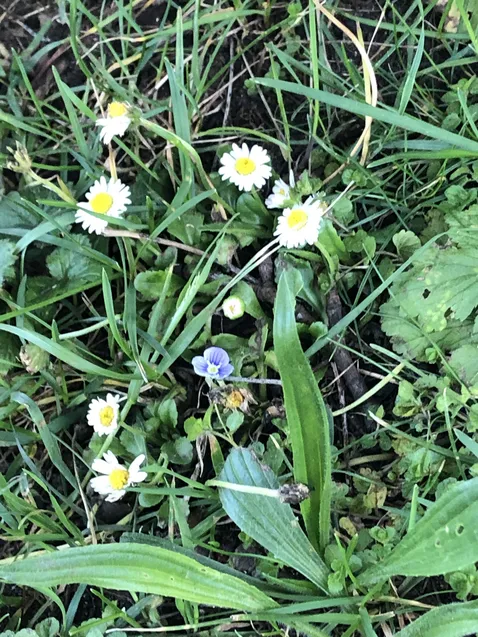
-
Kreuzer, A., Dalla Valle, L., and Czado, C. (2022)
A Bayesian Non-linear State Space Copula Model to Predict Air Pollution in Beijing
Journal of the Royal Statistical Society: Series C (Applied Statistics)
[link] -
Czado, C. and Scharl, S. (2021)
Analysis of an interventional protein experiment using a vine copula based structural equation model
[preprint] -
Barthel, N., Geerdens, C., Killiches, M., Janssen, P. and Czado, C. (2018).
Vine copula based likelihood estimation of dependence patterns in multivariate event time data.
Computational Statistics and Data Analysis, 117, 109–127.
[link] -
Nikoloulopoulos, A. K. (2017).
A vine copula mixed effect model for trivariate meta-analysis of diagnostic test accuracy studies accounting for disease prevalence.
Statistical Methods in Medical Research, 26(5), 2270–2286.
[link]
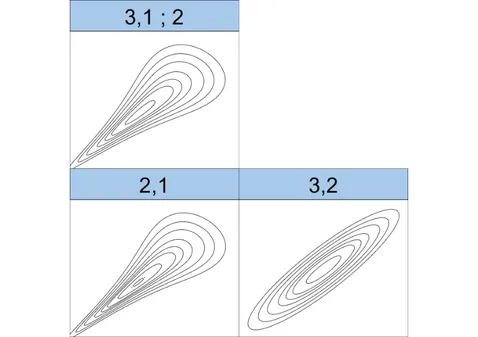
- Carrera, D., Bandeira, L., Santana, R. and Lozano, J. A. (2019)
Detection of sand dunes on Mars using a regular vine-based classification approach
Knowledge-Based Systems
[link]
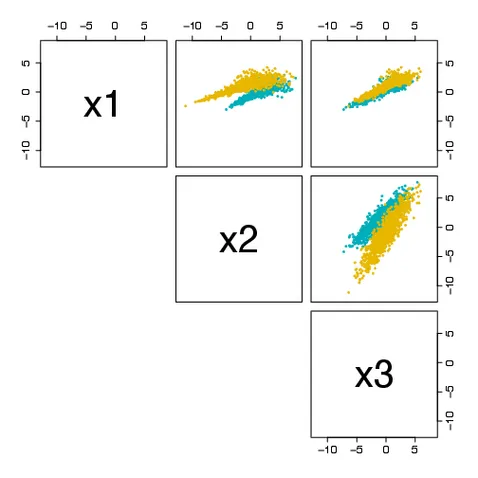
-
Sahin, Özge and Czado, Claudia (2022)
Vine copula mixture models and clustering for non-Gaussian data
Econometrics and Statistics
[preprint | link ] -
Sun, Mingyang, Ioannis Konstantelos, and Goran Strbac (2017)
C-Vine Copula Mixture Model for Clustering of Residential Electrical Load Pattern Data
IEEE Transactions on Power System
[link] -
Kim, Jong Min, Daeyoung Kim, Shu Min Liao and Yoon Sung Jung (2013)
Mixture of D-vine copulas for modeling dependence.
Computational Statistics and Data Analysis 64, pp. 1–19.
[link]

- Nagler, T., Krüger, D. and Min, A. (2022)
Stationary vine copula models for multivariate time series
Journal of Econometrics
[preprint, link] - Acar, E. F., Czado, C. and Lysy, M. (2019)
Flexible dynamic vine copula models for multivariate time series data
Econometrics and Statistics 12 (12), 181-197.
[link] - Schellhase, C. and Spanhel, F. (2018)
Estimating non-simplified vine copulas using penalized splines
Statistics and Computing, 28(2), 387–409.
[link] - Schamberger, B., Gruber, L. F. and Czado, C. (2017)
Bayesian inference for latent factor copulas and application to financial risk forecasting
Econometrics, 5(2), 21.
[link] -
Nagler, T. and Czado, C. (2016)
Evading the curse of dimensionality in nonparametric density estimation with simplified vine copulas
Journal of Multivariate Analysis, 151, 69–89.
[link]
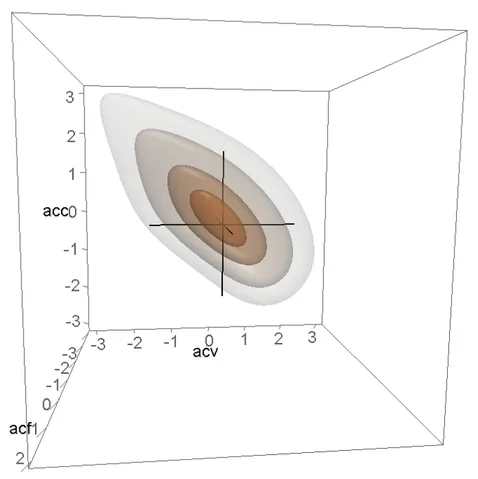
-
Nagler, T., Bumann, C. and Czado, C. (2019)
Model selection in sparse high-dimensional vine copula models with an application to portfolio risk.
Journal of Multivariate Analysis, 172, 180-192.
[link] -
Vatter, T. and Nagler, T. (2018)
Generalized Additive Models for Pair-Copula Constructions.
Journal of Computational and Graphical Statistics, 27(4), 715-727.
[link] -
Killiches, M., Kraus, D. and Czado, C. (2018)
Model distances for vine copulas in high dimensions.
Statistics and Computing, 28(2), 323–341.
[link] -
Schepsmeier, U. (2016).
A goodness-of-fit test for regular vine copula models.
Econometric Reviews, 1–22.
[link] -
Brechmann, E. C. and Joe, H. (2014).
Parsimonious parameterization of correlation matrices using truncated vines and factor analysis.
Computational Statistics and Data Analysis, 77, 233–251.
[link]
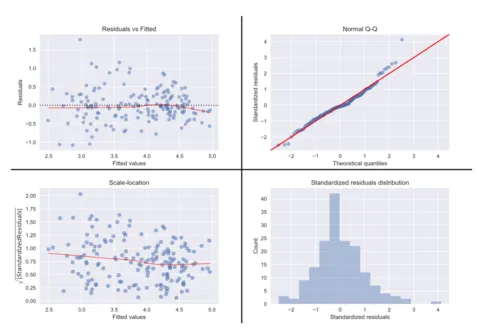
- Sahin, Ö. and Czado, C. (2022)
High-dimensional sparse vine copula regression with application to genomic prediction
[preprint] - Tepegjozova, M. and Czado, C. (2022)
Bivariate vine copula based quantile regression
[preprint] - Tepegjozova, M., Zhou, J., Claeskens, G. and Czado, C. (2022)
Nonparametric C- and D-vine based quantile regression
Dependence Modeling
[preprint | link ] - Chang, B. and Joe, H. (2019)
Prediction based on conditional distributions of vine copulas
Computational Statistics & Data Analysis [link] - Nagler, T., Vatter, T. (2018)
Solving estimating equations with copulas
arXiv:1801.10576 [preprint] - Kraus, D., and Czado, C. (2017)
D-vine Copula Based Quantile Regression
Computational Statistics and Data Analysis, 110, 1-18
[preprint] - Cooke, R. M., Joe, H. and Chang, B. (2015)
Vine Regression.
Resources for the Future - Discussion Paper, 15-52.
[link] - Schallhorn, N., Kraus, D., Nagler, T. and Czado, C. (2017)
D-vine quantile regression with discrete variables
submitted for publication
[preprint]
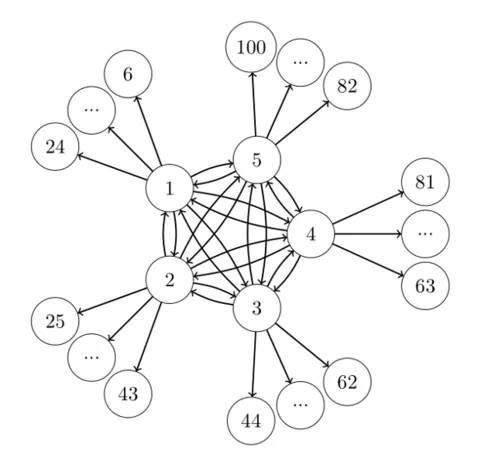
-
Krupskii, P., Huser, R. and Genton, M. G. (2018).
Factor copula models for replicated spatial data.
Journal of the American Statistical Association, 113(521), 467–479.
[link] -
Kraus, D. and Czado, C. (2017).
D-vine copula based quantile regression.
Computational Statistics and Data Analysis, 110C, 1–18.
[link] -
Schamberger, B., Gruber, L. F. and Czado, C. (2017).
Bayesian inference for latent factor copulas and application to financial risk forecasting.
Econometrics, 5(2), 21.
[link] -
Schallhorn, N., Kraus, D., Nagler, T. and Czado, C. (2017).
D-vine quantile regression with discrete variables.
[link]
2023
- Bax, K., Sahin, Ö., Czado, C., and Paterlini, S. (2023)
ESG, Risk, and (tail) dependence
International Review of Financial Analysis
[link] - Czado, C., Bax, K., Sahin, Ö., Nagler, T., Min, A., and Paterlini, S. (2023)
Vine copula based dependence modeling in sustainable finance
The Journal of Finance and Data Science
[link]
2022
- Sahin, Ö. and Czado, C. (2022)
High-dimensional sparse vine copula regression with application to genomic prediction
[preprint] -
Sommer, E., Bax, K., and Czado, C. (2022)
Vine Copula based portfolio level conditional risk measure forecasting
[preprint] -
Ye, F., Ding, J., Chen, K. and Xi, X. (2022)
Investigation of Corticomuscular Functional Coupling during Hand Movements Using Vine Copula
Brain Sciences
[link] -
Alnasser, H. and Czado, C. (2022)
An Application of D-vine Regression for the Identification of Risky Flights in Runway Overrun
[preprint] -
Tepegjozova, M. and Czado, C. (2022)
Bivariate vine copula based quantile regression
[preprint] -
Kreuzer, A., Dalla Valle, L., and Czado, C. (2022)
A Bayesian Non-linear State Space Copula Model to Predict Air Pollution in Beijing
Journal of the Royal Statistical Society: Series C (Applied Statistics)
[link] -
Sahin, Ö. and Czado, C. (2022)
Vine copula mixture models and clustering for non-Gaussian data
Econometics and Statistics
[preprint | link] -
Tepegjozova, M., Zhou, J., Claeskens, G. and Czado, C. (2022)
Nonparametric C- and D-vine based quantile regression
Dependence Modeling
[preprint | link ] -
Nagler, T., Krüger, D. and Min, A. (2022)
Stationary vine copula models for multivariate time series
Journal of Econometrics
[preprint, link] -
Yang, L. and Czado, C. (2022)
Two‐Part D‐Vine Copula Models for Longitudinal Insurance Claim Data
Scandinavian Journal of Statistics
[link] -
Czado, C. and Nagler, T. (2022)
Vine Copula Based Modeling
Annual Review of Statistics and Its Application, 9, 453-477
[link ]
2021
- Czado, C. and Scharl, S. (2021)
Analysis of an interventional protein experiment using a vine copula based structural equation model
[preprint] - Sahin, Ö., Bax, K., Paterlini, S. and Czado, C. (2021)
ESGM: ESG scores and the Missing pillar
[Preprint] - Kielmann, J., Manner, H. and Min, A. (2021)
Stock market returns and oil price shocks: A CoVaR analysis based on dynamic vine copula models
[link] - Bax, K., Sahin, Ö., Czado, C. and Paterlini, S. (2021)
ESG, Risk, and (tail) dependence
[preprint] - Coblenz, M. (2021)
MATVines: A vine copula package for MATLAB
[link] - Deng, Y. and Chaganty, N.R. (2021)
Pair-copula Models for Analyzing Family Data.
J Stat Theory Pract 15, 13.
[link][access]
2020
- Chang, B. and Joe, H. (2020)
Copula diagnostics for asymmetries and conditional dependence.
Journal of Applied Statistics, 47(9), 1587-1616.
[link] - Zhu, K., Kurowicka, D. and Nane, G. F. (2020)
Common sampling orders of regular vines with application to model selection
Computational Statistics & Data Analysis
[link]
2019
- Chang, B., Pan, S. and Joe, H. (2019)
Vine copula structure learning via Monte Carlo tree search
Proceedings of Machine Learning Research
[link] - Kreuzer, A. and Czado, C. (2019)
Bayesian inference for dynamic vine copulas in higher dimensions
[preprint] - Kreuzer, A. and Czado, C. (2019)
Efficient Bayesian inference for univariate and multivariate non linear state space models with univariate autoregressive state equation
[preprint] - Kreuzer, A., Dalla Valle, L. and Czado, C. (2019)
Bayesian Multivariate Nonlinear State Space Copula Models
[preprint] - Torre, E., Marelli, S., Embrechts, P. and Sudret, B. (2019)
A general framework for data-driven uncertainty quantification under complex input dependencies using vine copulas
Probabilistic Engineering Mechanics
[link]
2018
- Nagler, T., Bumann, C., Czado, C. (2018)
Model selection in sparse high-dimensional vine copula models with application to portfolio risk.
[preprint] - Vatter, T. and Nagler, T. (2018)
Generalized additive models for pair-copula constructions.
Journal of Computational and Graphical Statistics, to appear
[preprint] - Kreuzer A. and Czado C. (2018)
Bayesian inference for a single factor copula stochastic volatility model using Hamiltonian Monte Carlo
[preprint] - Barthel, N., Czado, C., and Okhrin, Y. (2018)
A partial correlation vine based approach for modeling and forecasting multivariate volatility time-series
[preprint] - Stübinger, J., Mangold, B., Krauss, C. (2018)
Statistical arbitrage with vine copulas
Quantitative Finance (published online) [link] [preprint] - Wilson, K. J. (2018)
Specification of Informative Prior Distributions for Multinomial Models Using Vine Copulas
Bayesian Analysis [link] - Barthel, N., Geerdens. C., Czado, C., and Janssen, P. (2018)
Dependence modeling for recurrent event times subject to right-censoring with D-vine copulas
[preprint]
2017
- Barthel, N., Geerdens, C., Killiches, M., Janssen, P. and Czado, C. (2017)
Vine copula based likelihood estimation of dependence patterns in multivariate event time data.
Computational Statistics & Data Analysis, doi.org/10.1016/j.csda.2017.07.010.
[link] - Schamberger, B., Gruber, L. and C. Czado (2017)
Bayesian Inference for Latent Factor Copulas and Application to Financial Risk Forecasting
Econometrics 2017, 5, 21; doi:10.3390/econometrics5020021
[pdf] - Kurz, M. and Spanhel, F. (2017)
Testing the simplifying assumption in high-dimensional vine copulas
submitted
[preprint] - Müller, D. and Czado, C. (2017)
Dependence Modeling in Ultra High Dimensions with Vine Copulas and the Graphical Lasso
submitted for publication
[preprint] - Kraus, D. and Czado, C. (2017)
Growing simplified vine copula trees: improving Dißmann's algorithm
submitted for publication
[preprint] - Killiches, M., Kraus, D. and Czado, C. (2017)
Model distances for vine copulas in high dimensions.
Statistics and Computing (doi:10.1007/s11222-017-9733-y)
[preprint] - Müller, D. and Czado, C. (2017)
Selection of Sparse Vine Copulas in High Dimensions with the Lasso
submitted for publication
[preprint] - Panagiotelis, A., Czado, C. Joe, H. and Stöber, J. (2017)
Model selection for discrete regular vine copulas.
Computational Statistics & Data Analysis, Volume 106, Pages 138–152
[preprint] - Christian Schellhase, Fabian Spanhel (2017)
Estimating Non-Simplified Vine Copulas Using Penalized Splines.
Statistics & Computing (doi: 10.1007/s11222-017-9737-7)
[preprint] - Müller, D. and Czado, C. (2017)
Representing sparse Gaussian DAGs as sparse R-vines allowing for non-Gaussian dependence
to appear in the Journal of Computational and Graphical Statistics (doi:10.1080/10618600.2017.1366911)
[link] - Fink, H., Y. Klimova, C. Czado J. and Stöber (2017)
Regime switching vine copula models for global equity and volatility indices
Econometrics 2017, 5, 3; doi:10.3390/econometrics5010003
[link][preprint] - Pereira, G., Veiga, A., Erhardt, T. M., and C. Czado (2017)
A periodic spatial vine copula model for multi-site streamflow simulation.
Electric Power Systems Research, 152, 9-17
[link] - Ivanov, E., Min, A. and F. Ramsauer (2017)
Copula-Based Factor Models for Multivariate Asset Returns
Econometrics 2017, 5, 20; doi:10.3390/econometrics5020020
[pdf] - M. Fischer, D. Kraus, M. Pfeuffer and C. Czado (2017)
Stress Testing German Industry Sectors: Results from a Vine Copula Based Quantile Regression
Risks 2017, 5(3), 38.
[preprint]
2016
- Vatter, T. and Nagler, T. (2016)
Generalized additive models for pair-copula constructions
Journal of Computational and Graphical Statistics, to appear
[preprint] - Killiches, M., Kraus, D. and Czado, C. (2016)
Examination and visualization of the simplifying assumption for vine copulas in three dimensions
Australian and New Zealand Journal of Statistics
[preprint] - Killiches, M., Kraus, D. and Czado, C. (2016)
Using model distances to investigate the simplifying assumption, goodness-of-fit and truncation levels for vine copulas.
submitted for publication
[preprint] - Prokhorov, A., Schepsmeier, U. and Zhu, Y. (2016)
Generalized Information Matrix Tests for Copulas.
under review in Journal of Multivariate Analysis - Schepsmeier, U. (2016)
A goodness-of-fit test for regular vine copula models.
Econometric Reviews (2016): 1-22.
[pdf] - Nagler T., Schellhase, C. and Czado, C. (2016)
Nonparametric estimation of simplified vine copula models: comparison of methods
Submitted
[preprint] - Nagler, T. and Czado, C. (2016)
Evading the curse of dimensionality in multivariate kernel density estimation with simplified vines.
Journal of Multivariate Analysis 151, 69-89 (doi:10.1016/j.jmva.2016.07.003)
[preprint] - Almeida, C., C. Czado and H. Manner (2016)
Modeling high-dimensional time-varying dependence using dynamic D-vine models
Applied Stochastic Models in Business and Industry 2016.
[link], [preprint] - Aas, K. (2016)
Pair-Copula Constructions for Financial Applications: A Review.
Econometrics 2016, 4, 43.
[link]
2015
- Sriboonchitta, S., Kosheleva, O. and Nguyen, H. T. (2015)
Why Are Vine Copulas So Successful in Econometrics?
[pdf] - Maya, R. A. L., Gomez-Gonzales, J. E. and Velandia, L. F. M. (2015)
Latin American Exchange Rate Dependencies: A Regular Vine Copula Approach
Contemporary Economic Policy, Volume 33, Issue 3, 535–549.
[link] - Cooke, R. M., Kurowicka, D., & Wilson, K. (2015)
Sampling, Conditionalizing, Counting, Merging, Searching Regular Vines
Journal of Multivariate Analysis 138, 4-18.
[link] - Spanhel, F. and Kurz, M. (2015)
Simplified vine copula models: Approximations based on the simplifying assumption
[preprint] - Schepsmeier, U. (2015)
Efficient information based goodness-of-fit tests for vine copula models with fixed margins.
Journal of Multivariate Analysis 138, 34-52.
(formerly: Efficient goodness-of-fit tests in multi-dimensional vine copula models)
[pdf] - Brechmann, E. and Joe, H. (2015)
Truncation of vine copulas using fit indices.
Journal of Multivariate Analysis 138, 19-33. - Gruber, L. and Czado, C. (2015)
Sequential Bayesian Model Selection of Regular Vine Copulas.
Bayesian Anal. Volume 10, Number 4 (2015), 937-963.
[preprint] - Stöber, J., Hong, H., Czado, C., Ghosh, P (2015)
Comorbidity of chronic diseases in the elderly: longitudinal patterns identified by a copula design for mixed responses.
Computational Statistics & Data Analysis, Volume 88, Pages 28–39.
[preprint] - Hobaek Haff, I. and Segers, J. (2015)
Nonparametric estimation of pair-copula constructions with the empirical pair-copula.
Computational Statistics & Data Analysis, Volume 84, Pages 1–13.
[pdf] - Bauer, A. and C. Czado (2015)
Pair-copula Bayesian networks
Journal of Computational and Graphical Statistics
[link] - Jaworski, P. (2015)
Univariate conditioning of vine copulas.
Journal of Multivariate Analysis, 138, 89-103.
[link] - Smith, M. and Vahey, S. (2015)
Asymmetric Density Forcasting of U.S. Macroeconomic Variables using a Gaussian Copula Model of Cross-Sectional and Serial Dependence.
[preprint] - Erhardt, T. M. and Czado, C. (2015)
Standardized drought indices: A novel uni- and multivariate approach
[preprint] - Erhardt, T. M., Czado, C. and Schepsmeier, U. (2015)
Spatial composite likelihood inference using local C-vines.
Journal of Multivariate Analysis 138, 74-88
[preprint][link] - Erhardt, T. M., Czado, C. and Schepsmeier, U. (2015)
R-vine Models for Spatial Time Series with an Application to Daily Mean Temperature.
Biometrics 71, 323-332
[preprint][link] - Killiches, M. and Czado, C. (2015)
Block-Maxima of Vines.
To appear in Extreme Value Modelling and Risk Analysis: Methods and Applications. Eds. D. Dey and J. Yan. Chapman & Hall/CRC Press.
[preprint] - Krupskii, P., & Joe, H. (2015).
Structured factor copula models: Theory, inference and computation.
Journal of Multivariate Analysis 138, 53-73.
[link]
2014
- E.C. Brechmann and H. Joe (2014).
Parsimonious Parameterization of Correlation Matrices Using Truncated Vines and Factor Analysis.
Computational Statistics & Data Analysis, Volume 77, Pages 233–251.
[preprint] [link] - Schepsmeier, U. and J. Stöber (2014)
Derivatives and Fisher information of bivariate copulas.
Statistical Papers, 55(2), 525-542.
online first: http://link.springer.com/article/10.1007/s00362-013-0498-x.
[pdf]
Web supplement: Derivatives and Fisher Information of bivariate copulas.
[pdf] - Stöber, J. and C. Czado (2014),
Detecting regime switches in the dependence structure of high dimensional financial data.
Computational Statistics & Data Analysis, 76, 672-686.
[link] - Min, A. and Czado, C. (2014),
SCOMDY models based on pair-copula constructions with application to exchange rates.
Computational Statistics and Data Analysis, 76, 523-535.
[link] - Smith, M. (2014)
Copula Modelling of Dependence in Multivariate Time Series.
International Journal of Forecasting, Volume 31, Issue 3, Pages 815–833
[link] [preprint] - Brechmann, E.C. and Czado, C. (2014),
COPAR - Multivariate Time Series Modeling Using the COPula AutoRegressive Model.
Applied Stochastic Models in Business and Industry, Volume 31, Issue 4, Pages 495–514.
[link] - Gräler, B. (2014)
Modelling Skewed Spatial Random Fields through the Spatial Vine Copula.
Spatial Statistics 10, 87 - 102.
[link] - E.C. Brechmann, C. Czado and S. Paterlini (2014),
Flexible Dependence Modeling of Operational Risk Losses and Its Impact on Total Capital Requirements.
Journal of Banking & Finance, 40, 271-285.
[pdf][link] - E.C. Brechmann (2014)
Hierarchical Kendall Copulas: Properties and Inference.
Canadian Journal of Statistics, 42(1), 78-108.
[link]
2013
- E.C. Brechmann, C. Czado and S. Paterlini (2013),
Modeling Dependence of Operational Loss Frequencies.
Journal of Operational Risk, 8(4), 105-126.
[link] - Brechmann, E.C., K. Hendrich and C. Czado (2013)
Conditional copula simulation for systemic risk stress testing.
Insurance: Mathematics and Economics, 53, 722-732
[pdf][link] - Brechmann, E.C. and C. Czado (2013),
Risk Management with High-Dimensional Vine Copulas: An Analysis of the Euro Stoxx 50.
Statistics & Risk Modeling, 30(4), 307-342.
[pdf] - Krupskii, P. and Joe, H. (2013).
Factor copula models for multivariate data,
Journal of Multivariate Analysis, 120, 85-101.
[pdf] - Krämer, N, Brechmann, E.C., Silvestrini, D. and Czado, C. (2013),
Total loss estimation using copula-based regression models
Insurance: Mathematics and Economics, 53, 829-839
[preprint][link] - Nikoloulopoulos, A. and Joe, H. (2013).
Factor copula models for item response data.
Psychometrika, 1-25.
[link] - E.C. Brechmann (2013)
Sampling from Hierarchical Kendall Copulas.
Journal de la Société Française de Statistique, 154(1), 192-209.
[link] - Stöber, J., H. Joe and C. Czado (2013),
Simplified Pair Copula Constructions - Limitations and Extensions
Journal of Multivariate Analysis, 119, 101-118
[pdf] - Lopez-Paz D, Hernandez-Lobato JM and Ghahramani Z (2013)
Gaussian Process Vine Copulas for Multivariate Dependence
In: JMLR W&CP 28(2): Proceedings of The 30th International Conference on Machine Learning, (Ed) S Dasgupta and D McAllester, 30th International Conference on Machine Learning (ICML 2013), JMLR, 10-18.
[pdf] - Dißmann, J., Brechmann, E.C., Czado, C., and Kurowicka, D. (2013)
Selecting and estimating regular vine copulae and application to financial returns.
Computational Statistics and Data Analysis, 59, 52-69
[pdf] - Brechmann, E.C., Czado, C. and Aas, K. (2012)
Truncated regular vines in high dimensions with applications to financial data.
Canadian Journal of Statistics, 40 (1), 68-85.
[pdf] - Czado, C., Jeske, S., & Hofmann, M. (2013).
Selection strategies for regular vine copulae.
Journal de la Société Française de Statistique, 154(1), 174-191.
[pdf] - Stöber, J. and U. Schepsmeier (2013)
Estimating standard errors in regular vine copula models
Computational Statistics, 28 (6), 2679-2707
[link] - E.C. Brechmann and U. Schepsmeier (2013),
Modeling Dependence with C- and D-Vine Copulas: The R Package CDVine.
Journal of Statistical Software, 52(3), 1-27.
[pdf] - Haff, I. H. (2013).
Parameter estimation for pair copula construction.
Bernoulli Journal, 19, 462-491
[pdf] - Kauermann, G. and Schellhase, C. (2013)
Flexible pair-copula estimation in D-vines with Penalized Splines.
Statistics and Computing, Volume 24(6), Pages 1081-1100 (doi: 10.1007/s11222-013-9421-5)
[link] - Schellhase, C. and Kauermann, G. (2013)
Flexible pair-copula estimation in R-vines for portfolio optimization.
working paper - Schmidl, D., Czado, C., Hug, S., and Theis, F. J. (2013)
A vine-copula based adaptive MCMC sampler for efficient inference of dynamical systems.
Bayesian Analysis, 8(1), 1-22
2012
- Acar, E., Genest, C. and Nešlehová, J. (2012)
Beyond simplified pair-copula constructions
Journal of Multivariate Analysis, 110, 74-90.
[link] - Czado, C., Schepsmeier, U., Min, A. (2012)
Maximum likelihood estimation of mixed C-vines with application to exchange rates
Statistical Modelling, 12 (3), 229-255
[pdf] - Panagiotelis, A., Czado, C. and Joe, H. (2012)
Pair copula constructions for multivariate discrete data.
J Amer Stat Assoc, 107:499, 1063-1072
[link] [pdf] - Bauer, A., Czado, C. and Klein, T. (2012)
Pair-copula constructions for non-Gaussian DAG models.
Canadian Journal of Statistics, 40 (1), 86-109
[pdf] - Nikoloulopoulos, A.K., Joe, H., and Li, H. (2012)
Vine copulas with asymmetric tail dependence and applications to financial return data.
Computational Statistics and Data Analysis, 56 (11), 3659-3673
[pdf] - Gräler, B. and Pebesma, E. (2012)
Modelling Dependence in Space and Time with Vine Copulas.
Presentation at: Geostats 2012, Oslo, Norway, 11-15 June 2012
[link] - Erhardt, V. and Czado, C. (2012)
Modeling dependent yearly claim totals including zero claims in private health insurance.
Scandinavian Actuarial Journal, 2, 106-129
[link] - Czado, C., Gärtner, F. and Min, A. (2011)
Analysis of Australian electricity loads using joint Bayesian inference of D-Vines with autoregressive margins
(Handbook on Vines, Editors: Dorota Kurowicka and Harry Joe, World Scientific)
2011
- Czado, C. and Min, A. (2011),
Bayesian Inference for D-vines: Estimation and Model Selection
Handbook on Vines, Editors: Dorota Kurowicka and Harry Joe, World Scientific. - Gräler, B. and Pebesma, E. (2011)
The pair-copula construction for spatial data: a new approach to model spatial dependency.
Procedia Environmental Sciences 7, 206-211.
[pdf]
2010
- Haff, I. H., K. Aas, and A. Frigessi (2010).
On the simplified pair-copula construction – simply useful or too simplistic?
Journal of Multivariate Analysis 101(5), 1296–1310.
[pdf] - Min, A. and C. Czado (2010).
Bayesian model selection for multivariate copulas using pair-copula constructions.
Journal of Financial Econometrics 8 (4), 511–546.
[pdf] - Hofmann, M. and Czado, C. (2010),
Assessing the VaR of a portfolio using D-vine copula based multivariate GARCH models
Submitted for publication.
[pdf] - Min, A. and C. Czado (2010).
Bayesian model selection for D-vine pair-copula constructions.
Canadian Journal of Statistics 39 (2), 239–258.
[pdf] - Smith, M., A. Min, C. Almeida, and C. Czado (2010).
Modeling longitudinal data using a pair-copula construction decomposition of serial dependence.
Journal of the American Statistical Association 105, 1467–1479.
[pdf] - Joe, H., Li, H. and Nikoloulopoulos, A.K. (2010)
Tail dependence functions and vine copulas.
Journal of Multivariate Analysis, 101, 252-270.
[pdf]